KI Absicherung - Safe AI for Automated Driving
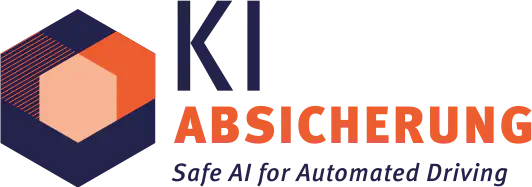
Environment perception can be seen as highly safety-relevant when it comes to automated driving. Deep neural networks (DNNs) are the state of the art in many computer vision disciplines. As a result, they are considered to be the go-to technology when it comes to environment perception. However, there are still concerns regarding the safety of DNN-based perception functions.
This is where the idea of KI Absicherung was born. This project addressed the question “How can we verify the safety of DNN-based functions?” in the context of automated driving. With a project budget of 41M € and a funding budget of 19.2M €, a consortium of 24 project partners from both industry and academia joined forces with additional 4 external partners and various subcontracted partners. From July 1st, 2019 until June 30th, 2022 the consortium developed methods and measures for the safety-aware application of deep neural networks.
I worked in very close cooperation with one of the large OEMs. During the course of the project, I developed and published several new concepts. I would like to thank the whole consortium and all my co-authors for the wonderful cooperation.
My publications as a first author:
A. Bär, M. Klingner, J. Löhdefink, F. Hüger, P. Schlicht, and T. Fingscheidt. Performance Prediction for Semantic Segmentation by a Self-Supervised Image Reconstruction Decoder, in Proc. of CVPR - Workshops, New Orleans, LA, USA, Jun. 2022, pp. 4399 - 4408.
A. Bär, J. Löhdefink, N. Kapoor, S. Varghese, F. Hüger, P. Schlicht, and T. Fingscheidt. The Vulnerability of Semantic Segmentation Networks to Adversarial Attacks in Autonomous Driving: Enhancing Extensive Environment Sensing, in IEEE Signal Processing Magazine, vol. 38, no. 1, pp. 42 - 52, Jan. 2021.
A. Bär, M. Klingner, S. Varghese, F. Hüger, P. Schlicht, and T. Fingscheidt. Robust Semantic Segmentation by Redundant Networks With a Layer-Specific Loss Contribution and Majority Vote (Best Paper Award), in Proc. of CVPR - Workshops, Virtual Conference, Jun. 2020, pp. 1348 - 1358.
My publications as a co-author:
S. Houben, S. Abrecht, M. Akila, A. Bär, F. Brockherde, P. Feifel, T. Fingscheidt, S. Gannamaneni, S. Ghobadi, A. Hammam, A. Haselhoff, F. Hauser, C. Heinzemann, M. Hoffmann, N. Kapoor, F. Kappel, M. Klingner, J. Kronenberger, F. Küppers, J. Löhdefink, M. Mlynarski, M. Mock, F. Mualla, S. Pavlitskaya, M. Poretschkin, A. Pohl, V. Ravi-Kumar, J. Rosenzweig, M. Rottmann, S. Ruping, T. Sämann, J. Schneider, E. Schulz, G. Schwalbe, J. Sicking, T. Srivastava, S. Varghese, M. Weber, S. Wirkert, T. Wirtz, and M. Woehrle. Inspect, Understand, Overcome: A Survey of Practical Methods for AI Safety, in Deep Neural Networks and Data for Automated Driving: Robustness, Uncertainty Quantification, and Insights Towards Safety, T. Fingscheidt, H. Gottschalk, and S. Houben, Eds. Cham: Springer Nature, 2022, pp. 3–78.
S. Varghese, C. Hümmer, A. Bär, F. Hüger, and T. Fingscheidt. Joint Optimization for DNN Model Compression and Corruption Robustness, in Deep Neural Networks and Data for Automated Driving: Robustness, Uncertainty Quantification, and Insights Towards Safety, T. Fingscheidt, H. Gottschalk, and S. Houben, Eds. Cham: Springer Nature, 2022, pp. 403 - 427.
N. Kapoor, A. Bär, S. Varghese, J. Schneider, F. Hüger, P. Schlicht, and T. Fingscheidt. From a Fourier-Domain Perspective on Adversarial Examples to a Wiener Filter Defense for Semantic Segmentation, in Proc. of IJCNN, Virtual Conference, Jul. 2021, pp. 1 - 8.
S. Varghese, S. Gujamagadi, M. Klingner, N. Kapoor, A. Bär, J. Schneider, K. Maag, P. Schlicht, F. Hüger, and T. Fingscheidt. An Unsupervised Temporal Consistency (TC) Loss to Improve the Performance of Semantic Segmentation Networks (Best Paper Award), in Proc. of CVPR - Workshops, Virtual Conference, 2021, pp. 12 - 20.
S. Varghese, Y. Bayzidi, A. Bär, N. Kapoor, S. Lahiri, J. D. Schneider, N. Schmidt, P. Schlicht, F. Hüger, and T. Fingscheidt. Unsupervised Temporal Consistency Metric for Video Segmentation in Highly-Automated Driving, in Proc. of CVPR - Workshops, Virtual Conference, Jun. 2020, pp. 1369 - 1378.
Source: https://www.ki-absicherung-projekt.de/en/.
Disclaimer: This text was written by Andreas Bär and only expresses his view on the project. This text does not reflect or imply any opinion of the consortium and its members.